Unlocking the Secrets of Explainable AI: A Necessary Evolution
Written on
Chapter 1: Understanding the Black Box of AI
What lies within the “black box”?
Modern large language models (LLMs) demonstrate remarkable capabilities. While they may not be as intelligent as some productivity enthusiasts suggest, their ability to generate art, music, and text is impressive. However, these generative AI models still face challenges, such as accurately depicting hands or producing coherent text with proper punctuation. Yet, they are improving rapidly, and it is fascinating to consider their potential advancements in just a few years.
A significant issue remains: we lack insight into how AI systems arrive at their decisions.
Oops! This predicament mirrors a very human trait: creating something powerful without understanding its inner workings.
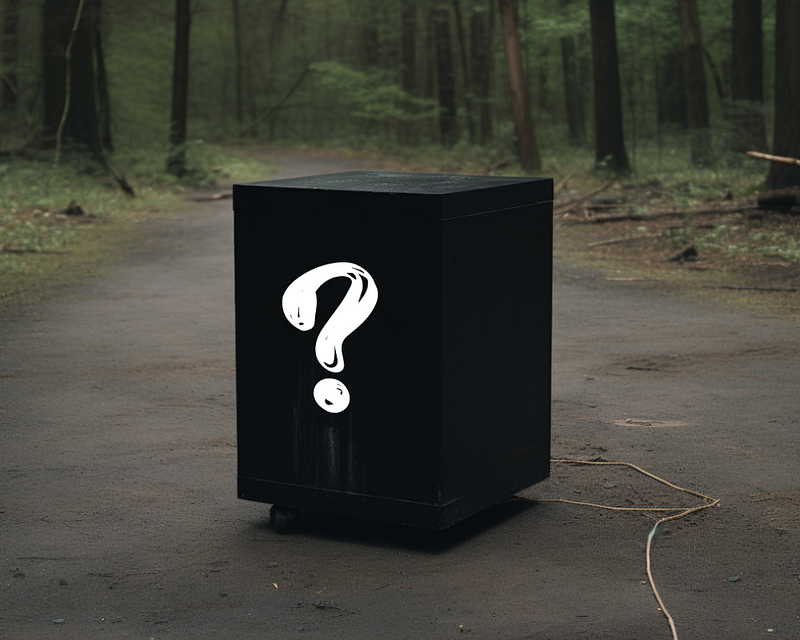
AI’s enigmatic “black box” dilemma. Image source: Investopedia
We simply input vast amounts of data, receive outputs, and hope for favorable results. Humans tend to be optimistic, after all! This situation is referred to as the “black-box” problem, and it is, indeed, a significant concern. To gain even a basic understanding of how AI “thinks” and reaches its conclusions, a new discipline within artificial intelligence has emerged: XAI (eXplainable Artificial Intelligence). This expansive field encompasses various tools and methodologies, making it a thrilling area to explore. Those dedicated to explainable AI are the modern-day heroes (minus the capes) striving to clarify the AI models that have become integral to our daily lives.
eXplainable Artificial Intelligence is essential to mitigate the numerous risks associated with daily AI usage. It also aids in identifying and addressing biases, data drift, and other inconsistencies within models. Currently, there are two primary approaches to achieving explainability in AI models:
- Designing inherently interpretable models: For instance, decision trees allow us to extract crucial insights into decision-making processes.
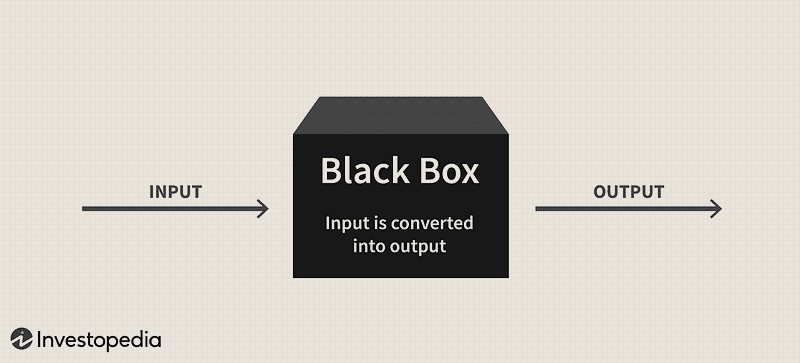
- Creating post-hoc explanations: This involves using techniques common in computer vision and image analysis to provide clarity after decisions have been made.
Why is this crucial?
I trust it’s clear how perilous it is to utilize third-party tools that arrive at conclusions independently, without elucidating their predictions, decisions, and actions. As companies around the globe increasingly adopt AI, this issue becomes even more pronounced. In my view, we often place excessive trust in new technologies. Each time I encounter a new AI-enhanced dating app, productivity tool, or self-help application, I can’t help but wonder:
Why do we assume AI can resolve all our issues?
Why do we rely on complex computer algorithms more than on our own judgment or on one another?
Implementing Explainable AI could foster trust and create more transparent AI models. Major tech companies, including Google, recognize the importance of this initiative (refer to the last link in Resources for further insights). They are actively developing tools that facilitate Explainable AI, such as the What-If Tool, which enables users to examine model behavior at a glance.
The first video titled The 6 Benefits of Explainable AI (XAI) highlights how XAI enhances accuracy, reduces harm, and improves storytelling in AI systems.
Resources:
- AI’s mysterious ‘black box’ problem, explained
- What is Explainable AI?
- Explainable AI Explained
Chapter 2: The Future of Explainable AI
The second video, Explainable AI Explained, delves into the core principles of Explainable AI, shedding light on its significance for future AI development.