Revolutionizing Robotics: The Power of Mind-Controlled AI
Written on
Chapter 1: Introduction to NOIR
At this point, my mother perceives certain AI technologies as almost magical. Today, we delve into one such remarkable innovation: NOIR. Developed at Stanford University, NOIR represents a groundbreaking model that enables individuals to manipulate robots using only their thoughts, a feat that is nothing short of extraordinary.
NOIR is designed as a versatile system capable of interpreting imagined movements through brain signals, translating them into actionable robotic motions. Thanks to advancements like NOIR, we can envision a future where individuals with physical disabilities can control their environments independently, leading fulfilling lives akin to anyone else.
But how does this technology actually function?
Most insights I share in my Medium articles have previously been discussed in my weekly newsletter, TheTechOasis. If you're eager to stay informed about the fast-paced world of AI while feeling motivated to engage with it, subscribing is a great way to keep ahead:
Subscribe | TheTechOasis
The newsletter to stay ahead of the curve in AI
thetechoasis.beehiiv.com
Chapter 2: Understanding the Mechanism of NOIR
A Tale of Two Components
To interpret brain signals, researchers opted for electroencephalography (EEG), which can be quite challenging to decode, especially when considering complex actions such as selecting an object visually, deciding on the method of interaction, and executing the action.
To address this complexity, NOIR is composed of two essential components: a modular goal decoder and a robotic system to carry out the tasks.
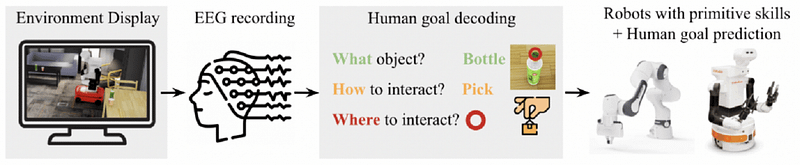
The modular decoder dissects the human intention into three critical elements: the object of interest, the method of interaction, and the location of interaction.
Focusing on the first aspect, NOIR utilizes SSVEP signals, a type of brain signal that corresponds to the flickering frequency of specific stimuli. The frequency refers to how often an event occurs within one second, measured in Hertz (Hz). For example, if I can jump three times in one second, my jumping frequency is 3 Hz.
In simpler terms, when you observe an object flickering at a particular frequency, your brain emits SSVEP signals that match that frequency. Thus, if multiple objects flicker at different frequencies, the one that correlates most strongly with the SSVEP signal will be the one that the individual is focusing on.
Traditionally, researchers have used LED lights to create flickering objects; however, Stanford's team employs Meta's DINO computer vision foundation model for simulation. When analyzing a scene, DINO segments all visible objects and applies a virtual flickering mask to each object, with each mask set to a slightly different flicker frequency for identification purposes.
This innovative technique allows researchers to determine which object a user is concentrating on.
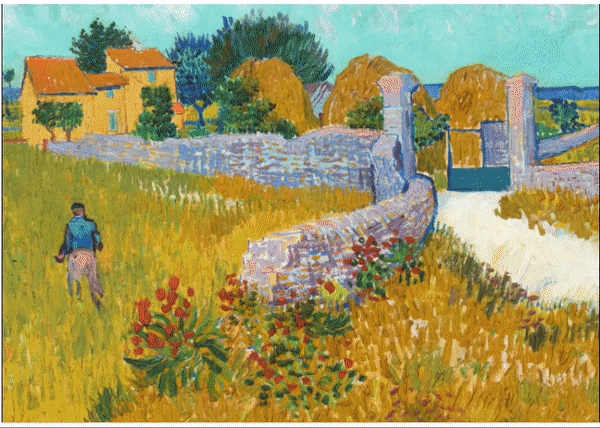
Next, to comprehend the intended action associated with the object, we need to address both the method and location, which introduces additional complexity.
From Imagination to Motion
When humans envision a movement, their thoughts generate brain signals known as Motion Imagery (MI). The challenge lies in accurately interpreting these signals to identify the specific action they represent.
Distinguishing between MI signals when a person thinks about "moving the left hand" versus the right hand is a complex task. In statistical terms, we aim to maximize the variance of a specific movement signal while minimizing the variance of other potential movements.
To achieve this, the researchers implemented the Common Spatial Pattern (CSP) technique, which identifies the components of a signal that display the highest variance relative to a specific movement.
To clarify this concept, consider an example where we analyze the brain signals of a person imagining two movements: moving the left hand (represented in red) and moving the right hand (represented in blue).
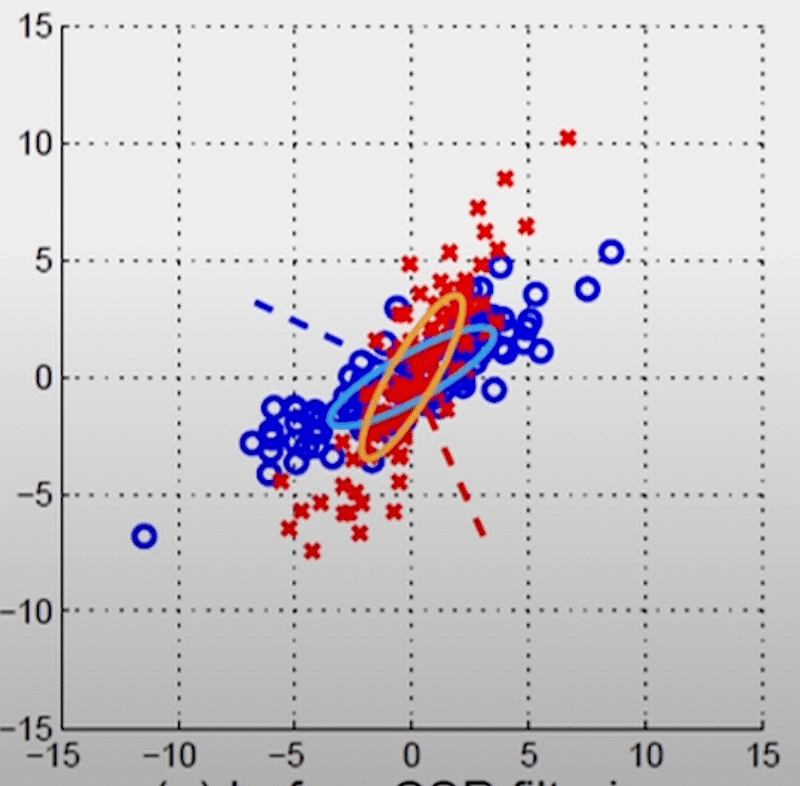
The signals are recorded across two brain channels at distinct frequencies, each represented by an axis on the graph. The brain emits signals at varying channels and frequencies, and here we focus on the 8–30 Hz range, where MI signals typically occur.
The graph indicates that the channel on the y-axis shows substantial variance concerning left-hand movement (red points), while there is minimal variance for the right-hand movement (blue points).
In statistical analysis, when a feature demonstrates high variance concerning a specific outcome, we can infer that it influences that outcome. If a channel indicates considerable variance for a particular movement, we can deduce that the individual may be thinking about that action.
However, it's essential to have a clear method for differentiation. After applying CSP filtering, the distinction becomes much clearer, as left-hand movement (red) is now distinctly influenced by the signals from the x-axis, while the opposite occurs for right-hand motion.
Next, researchers extract the most relevant features from each signal to delineate each movement accurately.
What does the complete process entail? The user views a specific environment and decides to perform an action, like "picking up a bowl and pouring water into a pan." While focusing on the bowl, NOIR segments the video frame and matches the SSVEP signal to the bowl's flickering mask.
Subsequently, the user imagines the action: moving the right arm to pick up the bowl. NOIR interprets the generated MI signals and identifies the intended movement. It then employs necessary functions, such as Pick(x,y) and Move(x,y), to instruct the robot on the required action.
Through this systematic approach, NOIR can execute various tasks—up to 20, marking it as a pioneering general-purpose Brain-Robot Interface and potentially the future of robotics.
This underscores the fact that AI fundamentally revolves around data transformation—organizing numerous variables and enabling them to learn a specific mapping for data transformation.
However, NOIR boasts an additional feature: adaptive behavior.
Adaptive Behavior: Learning from Experience
Despite NOIR’s impressive capabilities, decision-making, particularly in the MI realm, can be time-consuming. To enhance this aspect, researchers propose a method that enables the model to draw from past experiences to better interpret current actions.
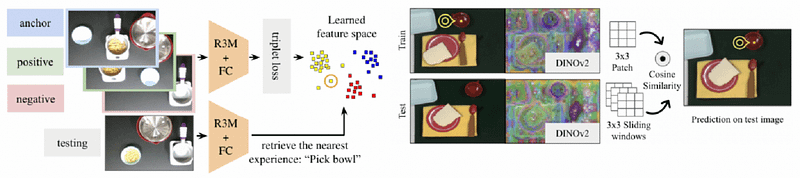
Utilizing image encoders, the model processes the environment into an image embedding—a vector representation of the image—placing it within a ‘feature space’ where similar images possess analogous vectors from prior experiences.
These past experiences encompass the object label and previously executed movements. For example, if the model recognizes a table with salt and steak, it processes the image, retrieves similar instances, and suggests that based on past scenarios, the logical action would be to pick up the salt and sprinkle it over the steak.
The outcome? A reduction of over 60% in decoding time and improved adaptability to user actions.
Chapter 3: The Future of AI and Robotics
NOIR undeniably brings us closer to enhancing the lives of individuals, such as quadriplegics, who yearn for autonomy over their lives. Although challenges remain on the path forward, the future appears promising for those facing significant obstacles to living a normal life—challenges that may soon become relics of the past.
The first video titled "Control Robots with Your Thoughts - Stanford NOIR" offers insights into the capabilities and implications of this technology.
The second video titled "Stanford Robot Learns from Humans" showcases how NOIR can adapt and learn from user interactions.